- 1University of Illinois at Urbana-Champaign
- 2Massachusetts Institute of Technology
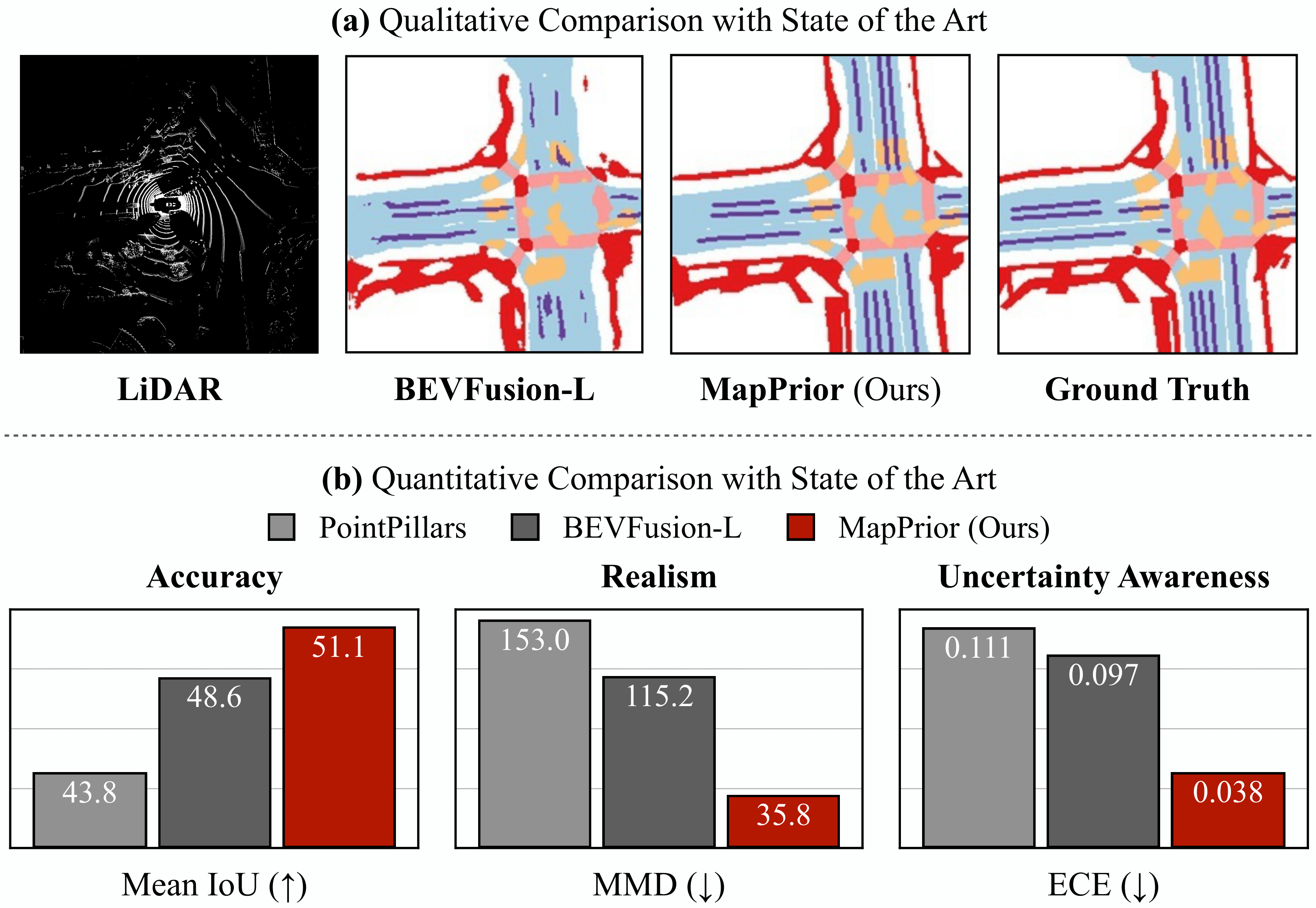
Abstract
Despite tremendous advancements in bird's-eye view (BEV) perception,
existing models fall short in generating realistic and coherent semantic map layouts,
and they fail to account for uncertainties arising from partial sensor information
(such as occlusion or limited coverage). In this work, we introduce MapPrior,
a novel BEV perception framework that combines a traditional
discriminative BEV perception model with a learned generative model for semantic map layouts.
MapPrior delivers predictions with better accuracy, realism and uncertainty awareness.
We evaluate our model on the large-scale nuScenes benchmark. At the time of submission, MapPrior outperforms the strongest competing method, with significantly improved MMD and ECE scores in camera- and LiDAR-based BEV perception. Furthermore, our method can be used to perpetually generate layouts with unconditional sampling.
We evaluate our model on the large-scale nuScenes benchmark. At the time of submission, MapPrior outperforms the strongest competing method, with significantly improved MMD and ECE scores in camera- and LiDAR-based BEV perception. Furthermore, our method can be used to perpetually generate layouts with unconditional sampling.
Bird's Eye View Map Estimation
* You can select different input modalities on different scenes and compare
our method with baselines (BEVFuison).
Modality
Scene
Diversity Sampling
Our method can sample multiple results per input with diversity, providing better uncertainty awareness:
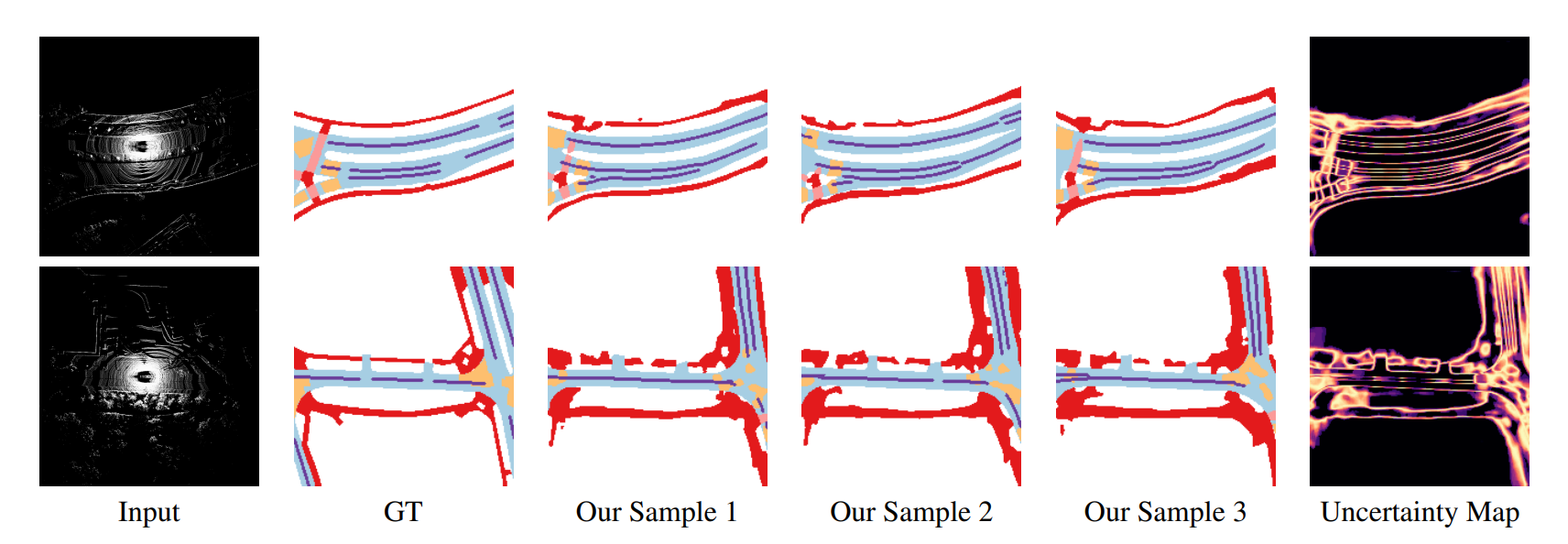
perpetual Generation
Our method can be exploited in a progressive manner to generate perpetual traffic layouts.
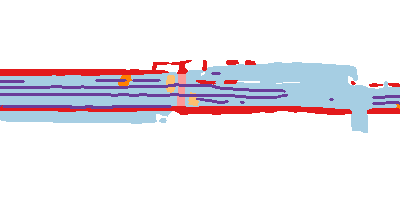
Map Estimation using Generative Models
MapPrior first makes use of an off-the-shelf perception model to generate an initial noisy estimate from the sensory
input, which uses monocular depth estimation to project camera features to BEV. It then encodes the noisy
estimate into a discrete latent code using a generative encoder and generates various samples through a transformer-based
controlled synthesis. Finally, MapPrior decodes these samples into outputs with a decoder
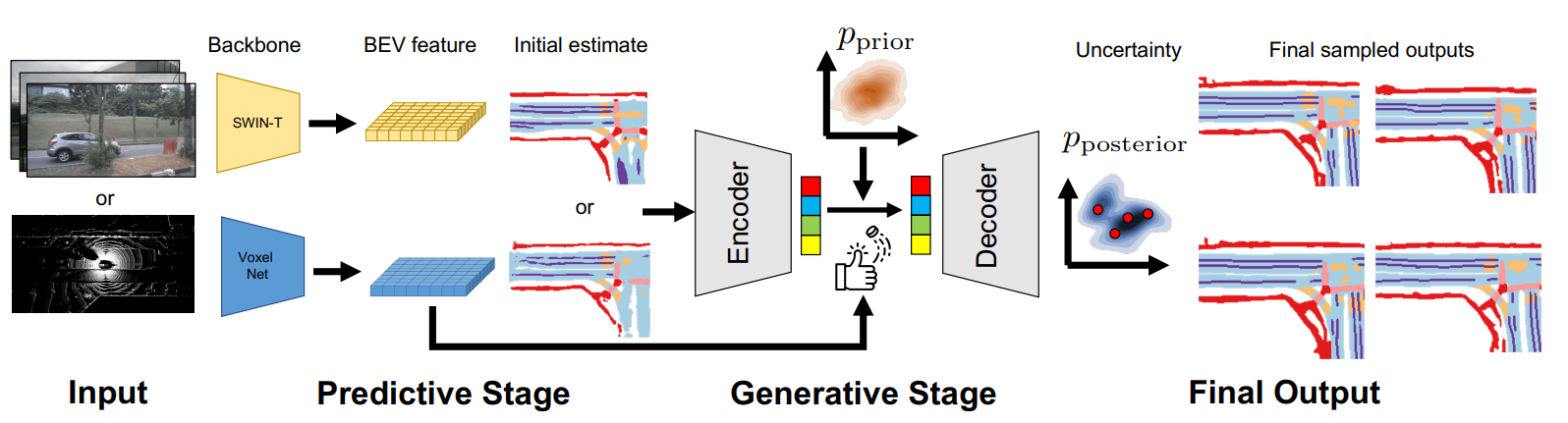
Quantitative Results
We show our quantitative metrics here. Our MapPrior achieves better accuracy (IoU), realism
(MMD) and uncertainty awareness (ECE) than discriminative BEV perception baselines.
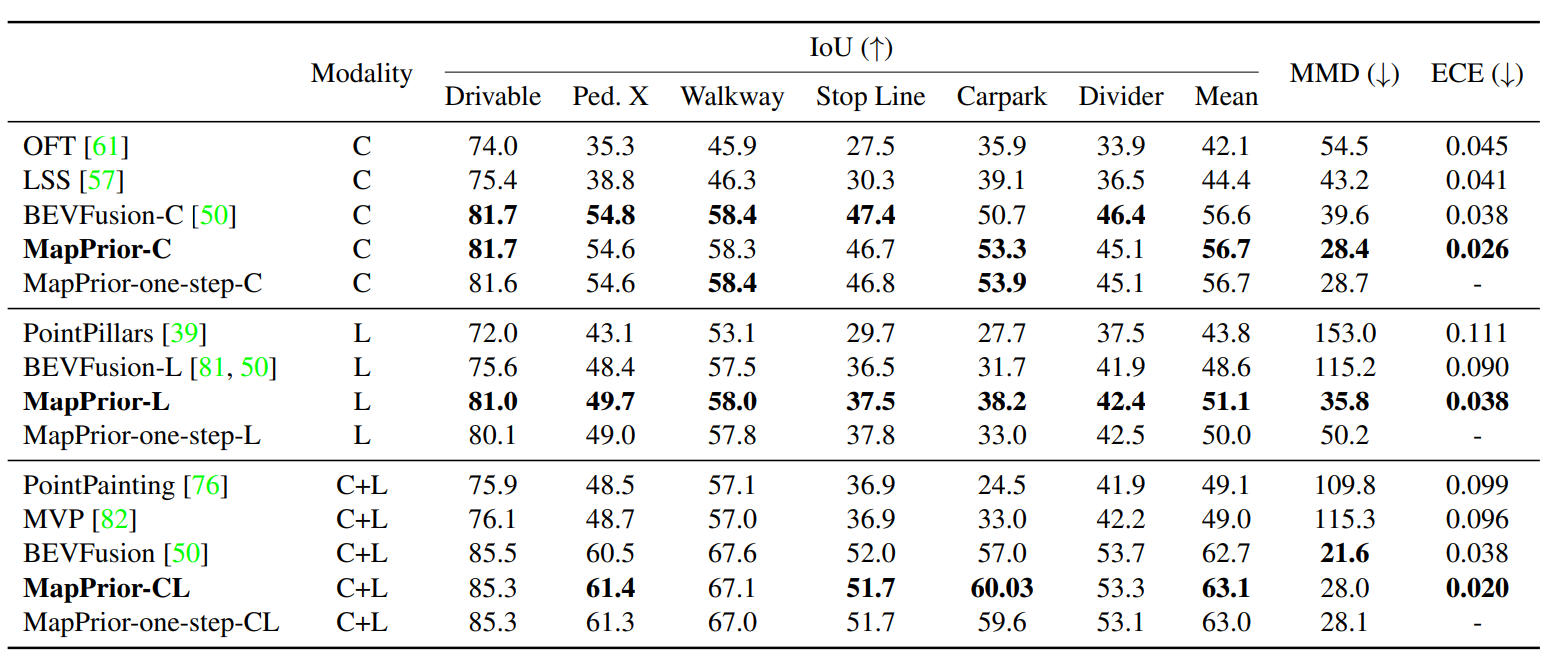